Can AI Really Predict Sports Injuries? The Truth About AI in Sports Medicine
- Carlos Jimenez
- Feb 17
- 5 min read
Introduction: The Rise of AI in Sports Medicine

Artificial Intelligence (AI) has revolutionized many industries, and sports medicine is no exception. With the increasing availability of wearable technology, advanced biomechanics assessments, and machine learning models, AI is now being used to analyze movement patterns, predict injuries, and optimize rehabilitation protocols. Sports organizations, medical professionals, and researchers are embracing AI as a tool to enhance injury prevention, player performance, and recovery strategies.
But can AI truly predict sports injuries with accuracy? Is it a reliable tool, or are we placing too much trust in technology? While AI offers significant benefits, it also comes with challenges such as data biases, ethical concerns, and over-reliance on algorithms over human expertise. This article explores the good, the bad, and the ugly of AI in sports medicine, revealing the truth behind its capabilities and limitations.
The Good: How AI is Transforming Injury Prevention
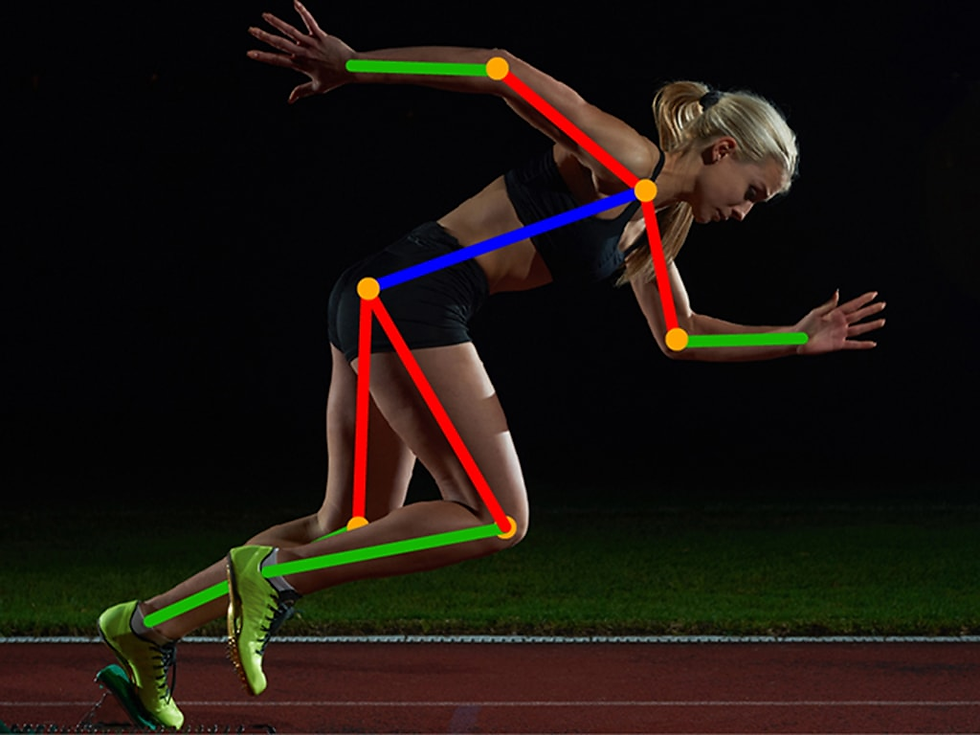
AI is rapidly changing how injuries are predicted and prevented in sports. Machine learning algorithms can process vast amounts of data from force plates, GPS trackers, and medical imaging to detect patterns that might indicate an increased risk of injury. Instead of relying solely on historical injuries or subjective assessments, AI enables data-driven decision-making that helps coaches and medical teams adjust training loads, movement patterns, and recovery protocols before an injury occurs.
One of the most exciting developments is AI-powered biomechanics analysis, which assesses joint angles, muscle activation, and force distribution during movement. This allows sports scientists to identify asymmetries or compensatory patterns that could lead to overuse injuries, such as ACL tears, hamstring strains, and stress fractures. Research has shown that AI can successfully flag high-risk athletes based on real-time monitoring, making injury prevention more proactive rather than reactive (Naughton et al., 2024; Ramkumar et al., 2021).
In addition to injury prediction, AI has also improved rehabilitation and return-to-play strategies. Athletes recovering from injuries can now be monitored using AI-driven movement tracking and force plate analysis, ensuring that their strength, flexibility, and neuromuscular control return to optimal levels before resuming competition. This reduces the likelihood of re-injury and allows for a more personalized recovery plan (Van Eetvelde et al., 2021).
The Bad: Limitations and Challenges of AI in Sports Medicine
While AI has made impressive strides, it is not a perfect system. One of the biggest limitations is the quality and diversity of data used to train AI models. Many injury prediction models rely on datasets that are heavily skewed toward elite male athletes, meaning that they may not generalize well to female athletes, youth sports, or recreational players (Reis et al., 2024). Since injury risks vary significantly based on gender, age, biomechanics, and playing conditions, relying on AI without addressing these biases can lead to flawed predictions.
Another issue is the black-box nature of AI models, where algorithms generate injury risk scores without fully explaining how they arrived at those conclusions. This lack of transparency makes it difficult for sports medicine professionals to trust AI
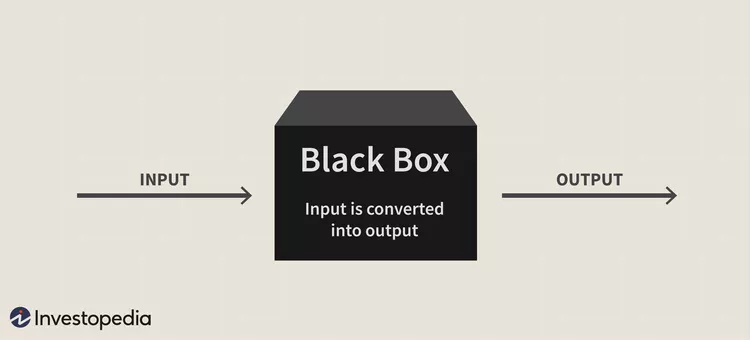
recommendations without additional validation (Munoz-Macho et al., 2024). If an AI system predicts that an athlete has a high injury risk but cannot justify why, should teams take that data seriously? This uncertainty can limit AI adoption and make it difficult for medical teams to integrate AI findings into clinical decision-making.
Moreover, AI is only as effective as the data it receives. Wearable technology, force plates, and motion tracking tools are expensive, making them inaccessible to lower-level teams, youth programs, and individual athletes. While professional sports teams can afford AI-driven performance monitoring, smaller organizations often lack the resources to implement AI at scale, reducing its broader impact on sports medicine (Mateus et al., 2025).
The Ugly: Ethical Concerns and Over-Reliance on AI
Beyond its technical limitations, AI in sports medicine also raises serious ethical concerns. Since AI systems collect biometric data, injury history, and movement tracking metrics, there is growing concern over data privacy and ownership. Who controls this sensitive information? Can teams use AI-generated injury risk scores in contract negotiations, salary decisions, or player evaluations? If not properly regulated, AI could be misused to justify benching players or limiting opportunities (Dandrieux et al., 2025).
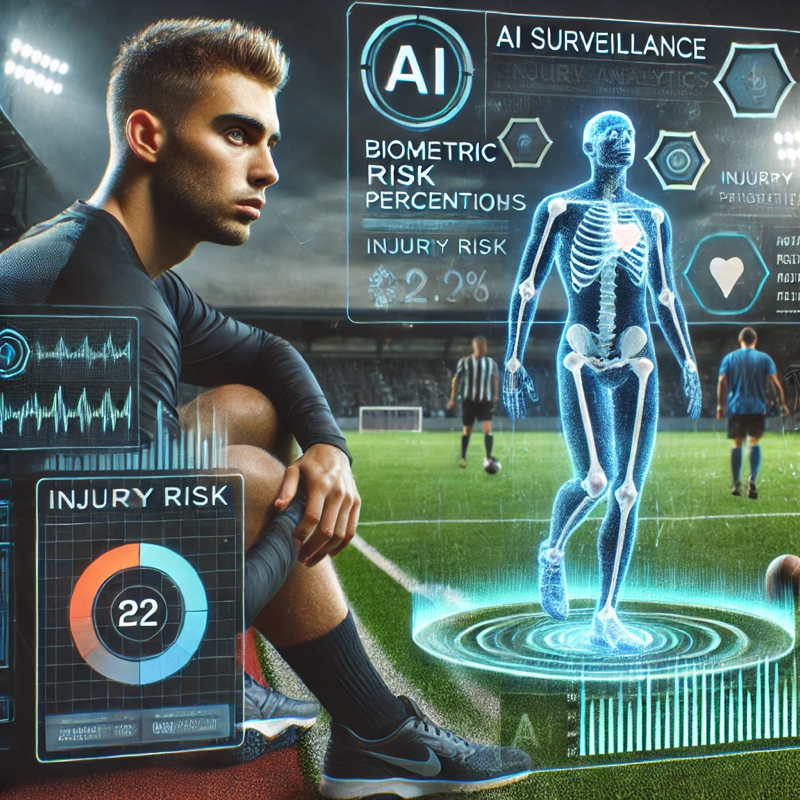
Another risk is the over-reliance on AI at the expense of human expertise. AI should complement—not replace—clinical reasoning and athlete feedback. There have been instances where AI incorrectly flagged an athlete as “high risk,” leading to unnecessary training restrictions despite the player showing no clinical symptoms (Musat et al., 2024). If teams and medical staff place blind trust in AI predictions, they may miss critical context that a trained sports medicine professional would otherwise recognize.
Additionally, AI-driven performance optimization tools might encourage athletes to push beyond safe limits, leading to overuse injuries rather than preventing them. While AI can optimize training workloads, it cannot fully account for mental fatigue, stress, or external pressures, which also play a role in injury prevention (Amendolara et al., 2023).
Final Thoughts: Should We Trust AI in Sports Medicine?
AI is here to stay in sports medicine, and its potential to prevent injuries, optimize performance, and aid rehabilitation is undeniable. However, AI is not a replacement for human expertise, and its effectiveness depends on high-quality data, ethical use, and careful interpretation.
So, can AI really predict sports injuries? Yes, but with limitations. AI can provide valuable insights, but it is not foolproof. The best approach is to use AI as a tool to support evidence-based sports medicine rather than relying on it as the sole decision-maker.
References
1. Amendolara, A., et al. (2023). An Overview of Machine Learning Applications in Sports Injury Prediction. Cureus.
2. Dandrieux, P.E., et al. (2025). Association Between the Use of Daily Injury Risk Estimation Feedback (I-REF) Based on Machine Learning Techniques and Injuries in Athletics (Track and Field). BMJ Open Sport & Exercise Medicine.
3. Mateus, N., et al. (2025). Empowering the Sports Scientist with Artificial Intelligence in Training, Performance, and Health Management. Sensors.
4. Munoz-Macho, A.A., et al. (2024). Performance and Healthcare Analysis in Elite Sports Teams Using Artificial Intelligence: A Scoping Review. Frontiers in Sports and Active Living.
5. Musat, C.L., et al. (2024). Diagnostic Applications of AI in Sports: A Comprehensive Review of Injury Risk Prediction Methods. Diagnostics.
6. Naughton, M., et al. (2024). Challenges and Opportunities of Artificial Intelligence Implementation within Sports Science and Sports Medicine Teams. Frontiers in Sports and Active Living.
7. Ramkumar, P.N., et al. (2021). Sports Medicine and Artificial Intelligence: A Primer. The American Journal of Sports Medicine.
8. Reis, F.J.J., et al. (2024). Artificial Intelligence and Machine Learning Approaches in Sports: Concepts, Applications, Challenges, and Future Perspectives. Brazilian Journal of Physical Therapy.
9. Van Eetvelde, H., et al. (2021). Machine Learning Methods in Sport Injury Prediction and Prevention: A Systematic Review. Journal of Experimental Orthopaedics.
Comments